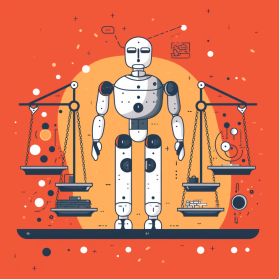
Large-scale class imbalance can adversely affect the performance of deep learning algorithms. To improve the model’s reliability, it is crucial to have strong generalizations on minority classes. In this research, we investigate reweighting the model loss function based on the sample characteristics to minimize a margin-based generalization bound. The novel re-weighting approach intends to be generic in nature so that it can be integrated with natural loss functions such as hinge loss. We also devise techniques to overcome the barriers to optimizing a reweighted loss function. We target to test the proposed framework on binary classification scenarios in cybersecurity-related applications: i.e., spam filtering and multi-class classification task with vision benchmark datasets.
Funding Body – NIPG Scheme
Investigators – Dr Charika Weerasiriwardhane (NIPG Fellow), Dr Suranga Seneviratne